Experiment in the age of data
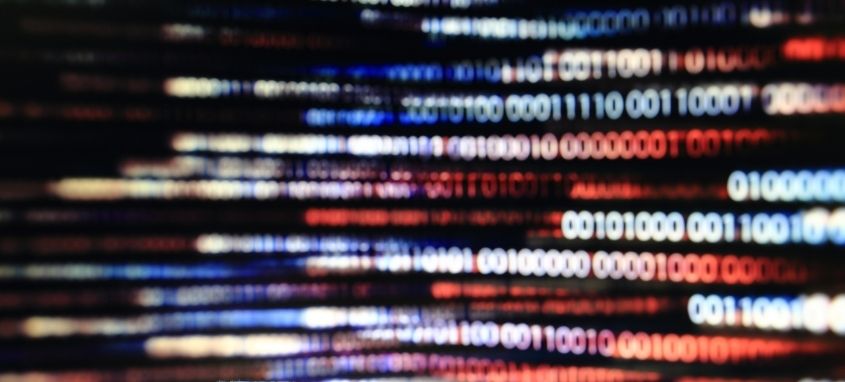
Relying on the current capabilities of data processing, many companies have placed continuous experimentation at the heart of their innovation strategy. How can you develop the rigor required for such an approach?
Carried by the technological progress of the latter years, an increasing number of companies rely on continuous experimentation to improve their offerings and operating modes. It is nowadays easy to collect masses of data to identify the highest performing variants in offerings, communication tools, tariffs, production processes, etc. We can also rely on inexpensive solutions to develop prototypes or virtual simulations of various user-experience modes.
This is how Expedia, Netflix or Google have built their business model. These companies conduct hundreds or even thousands of tests every day, with a genuine scientific rigor. When doing this, they are not looking for the next disruptive innovation: they bet on hundreds of small improvements to constantly perfect their product. At Microsoft Bing for example, daily improvements brought to the search engine have enabled an increase from 10% to 25% of the annual revenues per search for several years! This approach does not only concern online services: companies such as Wal-Mart, FedEx or H&M also use continuous experimentation to optimize their offering and develop their revenues.
These successes seem appealing, but they hide a trap: it is easy to extrapolate wrong conclusions from tests that are meant to be scientific. Biases are many and, of course, unconscious. This is how Petco pet shop chain tested its ideas in its best 30 outlets for years… before realizing that decisions were made on the basis of data that were not really representative of what was happening in other shops. Nowadays, the company makes sure it chooses its testing stores randomly, verifying that they are representative of the whole network in terms of size, demographics and localization.
Experimentation has its full place in a strategy based on continuous optimization. But it demands a great vigilance on how experiences are built and their results interpreted. Here are a few pieces of advice to this effect.
In this synopsis:
– Avoiding the main interpretation biases of quantitative data
– Developing a culture of continuous experimentation
– Conducting an A/B test with rigor
SubscriberSign in
to download
the synopse (8 p.)
VisitorI want to buy
this synopsis (8 p.)
VisitorI want
to subscribe
See also
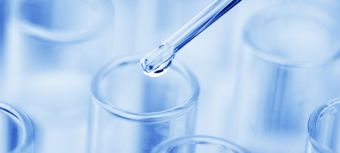
Innovate through experimentation
Experimentation is a powerful innovation accelerator which, thanks to the digital revolution, is much more accessible than before. Yet it remains under-utilized. How can you prompt people to acquire the reflex to experiment with their ideas?
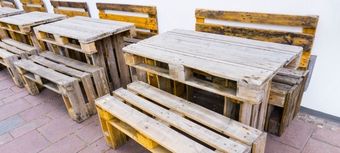
Reconnect with your ingenuity to boost your capacity for innovation
Long considered as players with low added value, emerging countries are showing a surprising ability to innovate. What can be learned from their innovation approach?
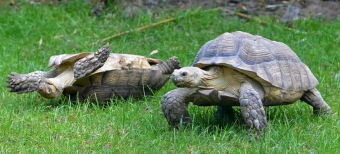
Handling failures productively
Accepting the possibility of failure is essential in order to be able to experiment, innovate and adapt. But on a day-to-day basis, it is very often the need for operational performance that prevails. How can we identify and promote productive failures?