Management gemsFind here some gems from our monitoring of the best publications on leadership and management
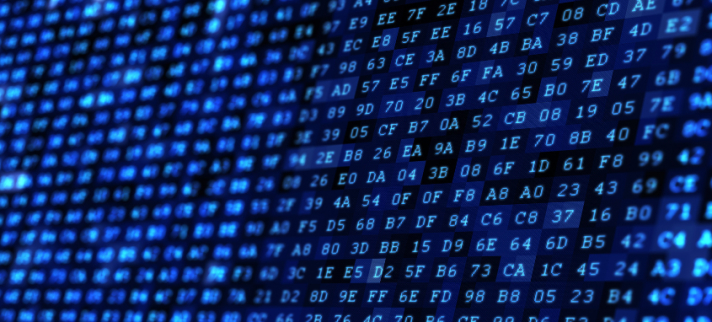
How can we avoid passing on discriminatory biases to our algorithms?
In 2023, eight out of every ten companies planned to invest in machine learning. This sub-field of artificial intelligence permits the detection of recurring patterns within data to guide decision-making.
Many decisions can thus be delegated to algorithms: selecting among candidates for a recruitment, for a loan… But how can we educate our algorithm to avoid biases, and in particular discriminatory ones? Experimentations have indeed shown that AI risks amplifying discriminations already in play. This results from the fact that it relies on selection histories to carry out its training—histories that are often biased and lead to certain populations going under-represented.
In rather counter-intuitive fashion, a study on a credit-management algorithm suggests that sharing sensitive personal data with it, rather than masking this data during its training, allows to meaningfully reduce the risk of discrimination. Cherry on top: the profitability of the loans granted by this algorithm also increased by 8%. When it isn’t possible to include this data directly in the algorithm’s training phase, corrective factors can be applied to rebalance the samples it receives, for instance by increasing the share of traditionally under-represented populations.
Source: Removing Demographic Data Can Make AI Discrimination Worse, Stephanie Kelley, Anton Ovchinnikov, Adrienne Heinrich, David R. Hardoon, Harvard Business Review, March 2023.